Cardano Mainnet: Pledge Influence Factor Analysis
Summary:
With the launch of Cardano mainnet around the corner, we have seen a spike of interest around the pledge influence factor (a)—an important element in Cardano’s reward scheme. The pledge influence factor has always been a part of the Cardano specifications, but its implementation was omitted in Cardano ITN testnet.
In this paper we outline our understanding of how pledge influence factor works and examine its implications on the decisions of stake pool operators and Cardano stakeholders. More specifically, we examine the reward distribution formula outlined in the research paper “Reward Sharing Schemes for Stake Pools” by Lars Brünes, Aggelos Kiayias, et al. and analyze its behavior under a range of possible scenarios.
Finally, we present our findings in terms of their importance to stake pool operators and stakeholders alike. Here are our key observations:
- The goal of the pledge influence factor is to introduce the concept of “skin in the game” for Cardano stake pool operators and protect the network against the sybil attacks.
- The pledge factor achieves that by reducing the rewards for pools with little or no pledge
- The severity of the reward penalty depends on the level of the pledge influence factor. The higher pledge influence factor the more severe reward penalties for pools with lower pledges.
- Pool Operators: The amount of pledge required to maximize rewards depends on the k factor. With k factor at 1000, the amount of pledge that would generate maximum rewards equals approximately 32M ADA. The marginal rewards between a high-pledge and zero-pledge pool is highly dependent on the value of the pledge influence factor. The annualized ROS difference between a pool with zero pledge vs a pool with a pledge of 3M ADA is between 0.03% to 1.04% for pledge influence factor values in the range of 0.02 to 1.5.
- We expect the pledge influence factor to be set between 0.15 and 0.75 for the mainnet and outline our support for this in our analysis below.
- Stakeholders: All-else equal, the stakeholders will maximize their rewards by delegating to stake pools with higher pledge amounts. However, they are advised to look at their net performance to compare pools as differences in pool performance may offset the gains obtained by delegating to higher pledged pools. It is a balancing act.
What is Pledge Influence Factor and Why Does it Matter?
Unlike other proof-of-stake networks, Cardano does not have the concept of minimum pledge. Instead, it uses something called a pledge influence factor to secure its network against sybil attacks. The pledge influence factor is a system constant that effectively penalizes pools with low pledge amounts by lowering their staking rewards.This incentivizes the stakeholders to avoid such pools as they will yield the lowest returns.
The pledge influence factor is part of the reward equation, which calculates the amount of rewards each stake pool receives at the end of each epoch. The formula first appeared in the IOHK paper Reward Sharing Schemes for Stake Pools and is now part of the design specification for the Cardano mainnet.

- R=total stake
- k=desired number of pools [a system constant]
- a=pledge influence factor [a system constant]
- ơ'=pool’s share of the total stake, capped at 1/k
- ƛ’=share of pledge in total stake, capped at 1/k
- b=maximum pool size = 1/k
Reward penalties impose real costs to rogue actors who may otherwise create multiple pools and gain enough stake to attack the Cardano network. The expectation here is that the performance penalties would be large enough to discourage stakeholders from delegating their stake to the stake pool operators with little or no skin in the game.
What Is the Ideal Pledge Amount?
Since there are no minimum pledge amounts in Cardano, the pool operators are free to pledge any amount. The reward calculations benefit pools with largest pledge amounts. These pools will always earn better returns than comparable pools with lower pledge amounts.
This incentivizes stake pool operators to pledge all their personal stake into one pool. As we demonstrate below, the size of the extra rewards due to the increase in pledge amount depends on the absolute level of the pledge influence factor. At lower levels, the difference in returns between a pool with a 3M ADA pledge and a pool with zero pledge is negligent, but this difference grows significantly at higher pledge influence factors.
The Analysis
In this section we analyze the effect of the pledge influence factor on the outcome of rewards for a stake pool. We do this by isolating this factor, changing its value and recording our observations. The purpose of this exercise is to home in on a narrower range of values for the pledge influence factor. We also analyze the effect of (i) changing the pledge amount and (ii) the total pool stake on rewards.
Any meaningful analysis of a complex system requires that we make certain assumptions about the factors that determine the outcomes that are of interest to us. Cardano is a prime example of such a system.
At this point, we do not know the exact values of some critical components (i.e. total rewards, k value) that determine pool rewards. Nevertheless, we can make reasonable assumptions on some of these and analyze outcomes under a range of conditions. Below is our list of main assumptions. These form the basis for our analysis. Again, we fix these in order to isolate the effect of pledge and total pool stake amount on total pool returns.

Desired number of pools: This number reflects our expectation on the number of pools in Cardano mainnet.
Inflation: We picked a number that would be competitive compared to other protocols. We understand that the calculation of rewards follows a decaying emission schedule.
Staking Ratio: In order to keep analysis simple, we keep the staking ratio to 100%.
Total Liquid Supply: This is the amount of ADA in circulation now plus the estimated amount of ITN staking rewards.
How Does the Pledge Influence Factor Affect Returns?
Pledge influence works by introducing return penalties to pools with low pledged amounts. The steepness of these penalties depends on the value of the pledge influence factor. The higher the pledge factor the greater the return gap between top and bottom pools. The table below illustrates the concept. The columns in this table represent the amount of the pledge the rows are the values for the pledge influence factor a.

For this example, we take a saturated pool and change the amount of pledge and the pledge influence factor. This table shows us how the amount of pledge improves the annual returns. The extent of this improvement is dependent on the pledge influence factor as illustrated the series of charts below.

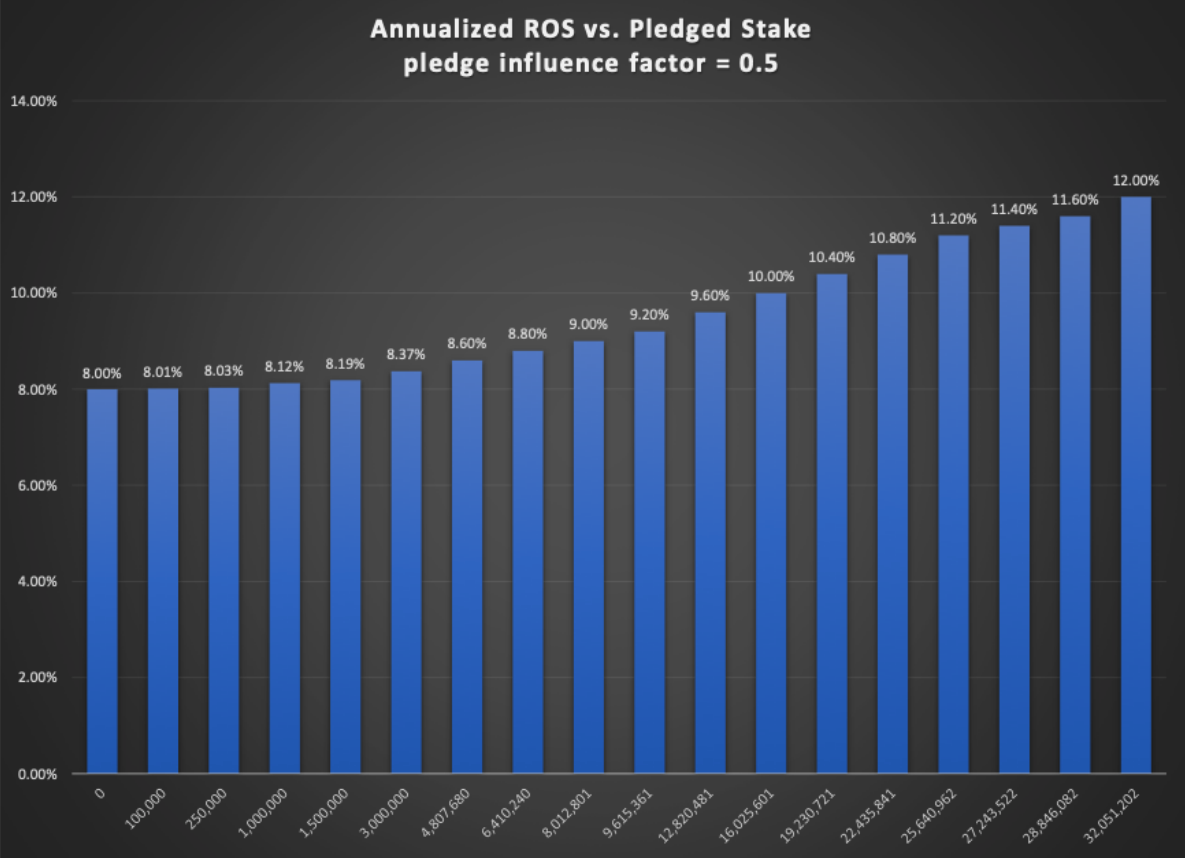
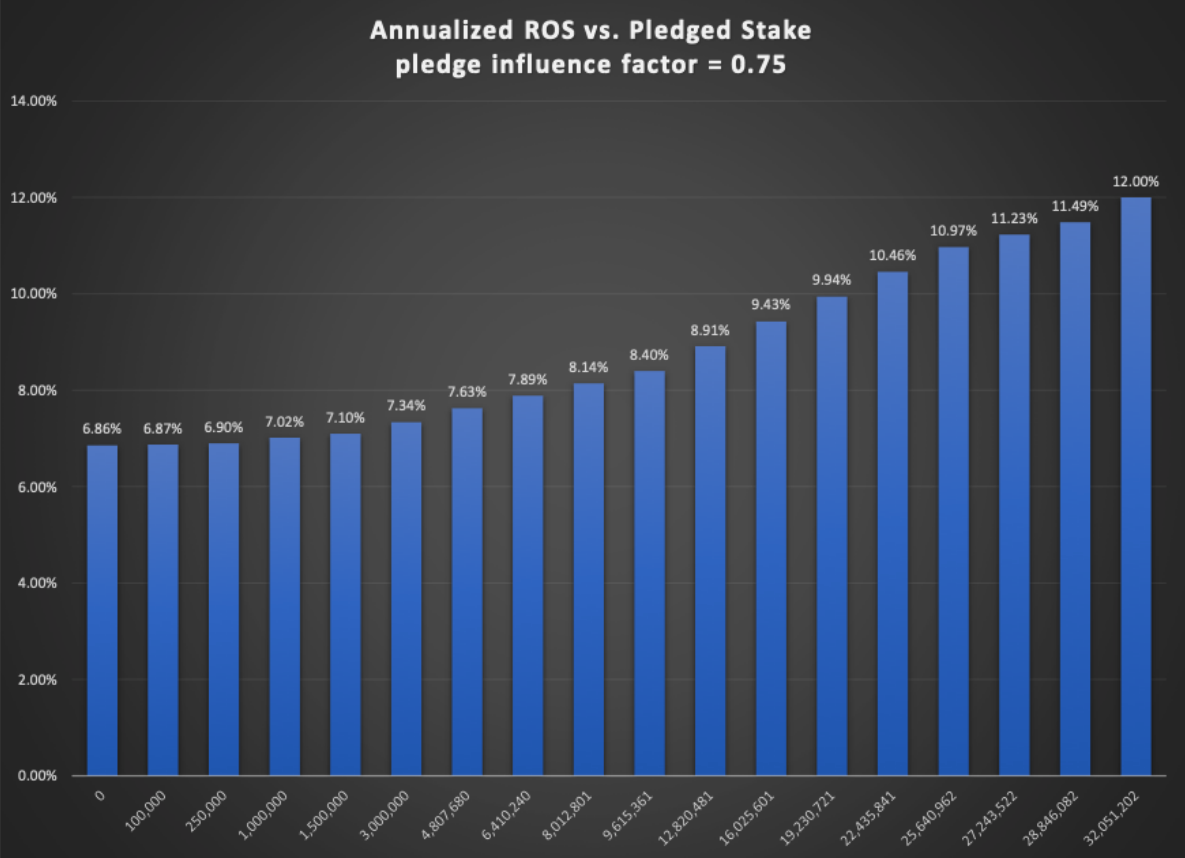
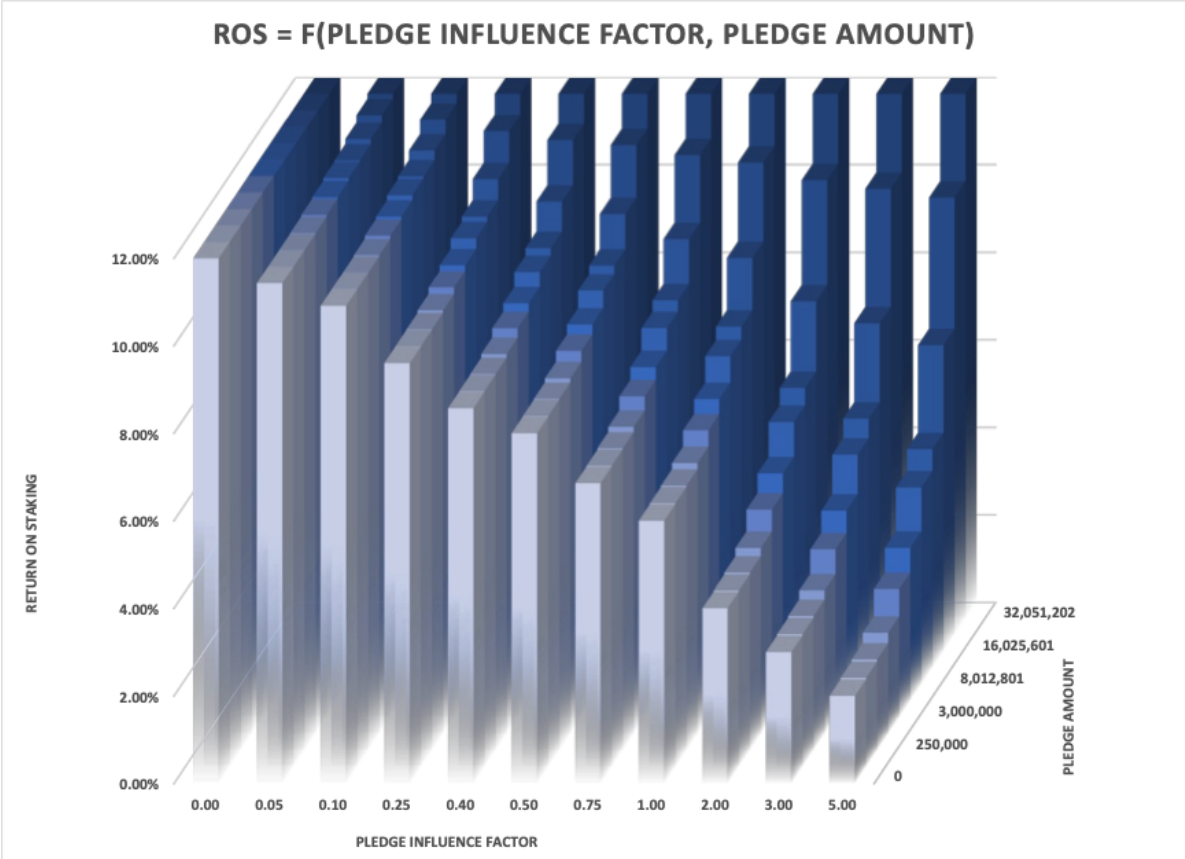
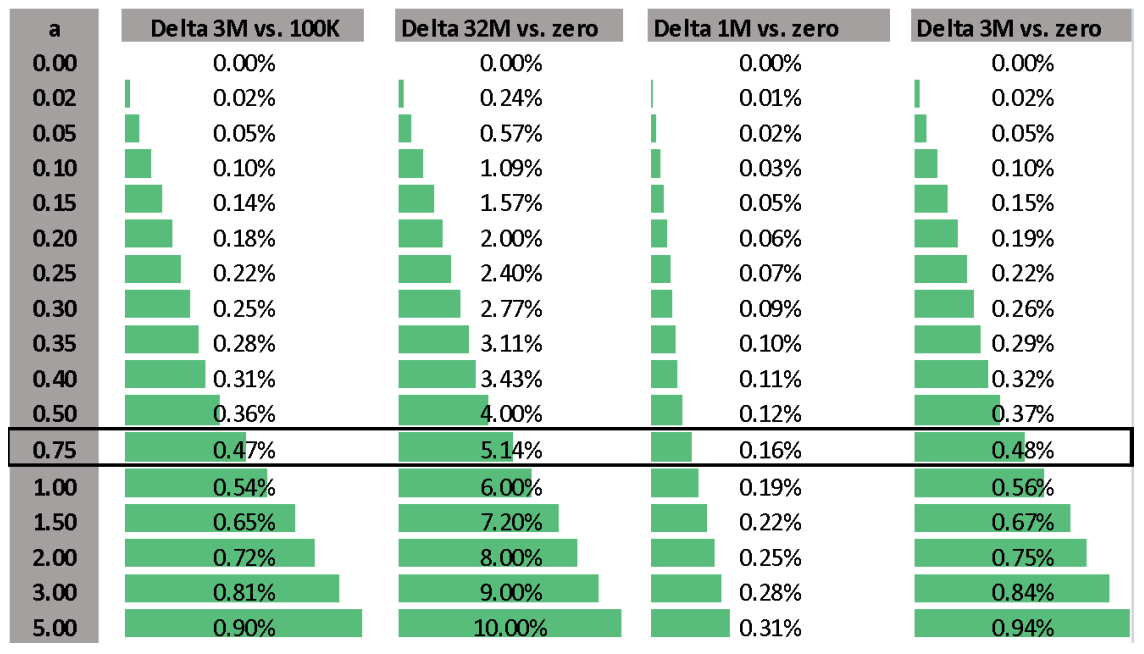
The table above shows the amount of extra return a stake pool generates for its stakeholders by increasing its pledge contribution. At low levels of pledge influence factor this difference is negligible but increases steeply as we progress toward higher numbers. For example, at pledge influence factor = 0.75, a saturated pool with 1M ADA pledged to it generates 0.16% extra per annum.
Given a pledge amount of [500K ADA] how does the total pool size influence returns?
Pool operators and stakeholders may want to know how the returns change in response to the amount of stake for a pool with a certain stake. The table below illustrates return paths for a pool with a 500K ADA pledge. Higher pledge influence factor introduces a gap between maximum returns (12%) and achievable returns. For example, with pledge influence factor set at 0.75, a stake pool with a 500K ADA pledge can earn anywhere from 6.86% to6.94%. The size of the pledge locks a pool into a return tier with known minimum and maximum potential returns.
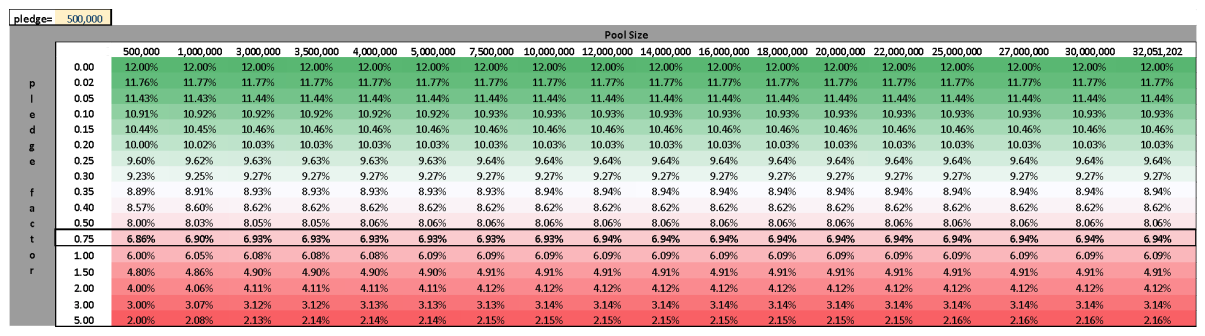
Regardless of pledge influence factor level, a pool with a zero pledge will always have the lowest return. Moreover, the pools with zero pledges do not enjoy increased returns as they get more stakeholders. The pools with non-zero pledges and no delegators will also start at the lowest return but increase their return as they gain stakeholders. However, this is not always the case. Pools with at least 1.5M ADA pledged to them have higher minimum returns than the pools with lower pledges.
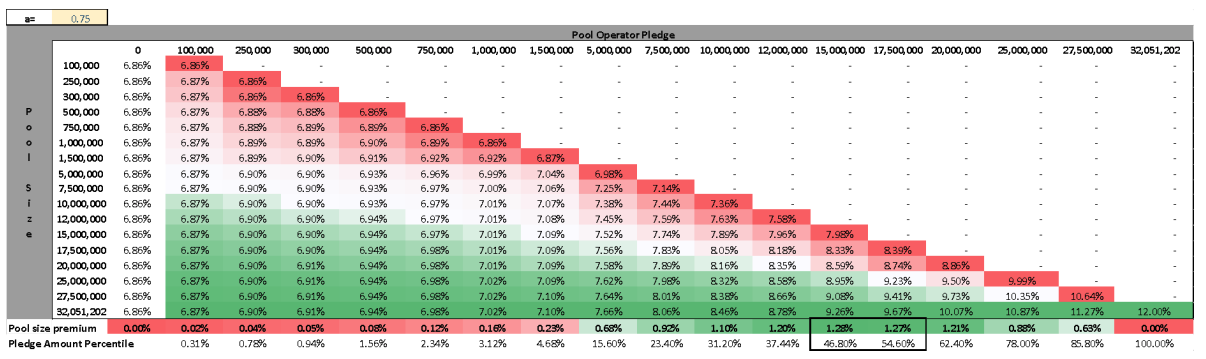
This threshold is dependent on the level of pledge influence factor. For example, at a=1 it moves to 1M ADA as illustrated below.

The amount of stake over and above the pledge amount increases returns slightly creating some overlap between pools. For example, a saturated pool with 500K ADA pledged to it generates a higher return than a pool with 1M ADA and no delegators. However, the pool with a 1M ADA pledge will always have the potential (i.e. a runway) to generate higher returns as it acquires more stake.
Pools that have pledges at 50% of saturation point, maximize their return from the growth in pool size. The absolute maximum return is only earned by pools with pledge amount equal to the pool saturation point.
At what level should the pledge influence factor be set?
That is a million-dollar question! This crucial factor should be set a level that would protect the network against sybil attacks while making sure that pools with smaller pledge amounts are not permanently penalized. How do we determine that sweet spot?
Method 1: The answer depends on how much ADA is available to most pool operators to commit as a pledge, and on their minimum fees. Let us say that number is 1M ADA and most pools are fine charging 1% fees. Knowing this allows us to determine the level of pledge influence factor that would make it economically attractive for stakeholders to choose these pools over potentially rogue pools with zero-pledge and zero fees.

The table above compares the return delta between a 1% pool with a 1M ADA pledge and a zero fee, zero-pledge pool. The right column contains the pool costs expressed as a total percentage return. Highlighted cells mark the range of pledge influence factors that would make it economically more attractive to delegate to a 1M ADA pool with 1% fees than to delegate to a zero-pledge poo with zero fees.
Ideally, we would pick 0.35 here as it produces the highest overall return for pools. This exercise can be repeated for different pledge and fee levels using our excel model [available upon request].
Method 2: Have IOHK, CF and Emurgo sign contracts with several reputable pool operators to fund their pledge accounts up to some higher threshold amount (say 3M ADA?). This would require around 1.5B ADA to earmark to 501 honest pools and would augment the security of the network, improve decentralization while increasing the overall returns for stakeholders.
Of course, this does not have to be limited to the three entities above. Realistically, anyone with enough funds could fund the pledge account of their preferred pool and earn extra rewards. Doing so would allow Cardano to set the pledge influence factor at 0.15.

Conclusion
The science and art of setting the pledge influence factor requires a careful balance between network security, availability of funds for stake pool operators and overall returns. Given the above we think this factor is likely to be set between 0.15 and 0.75.
All else equal, to maximize returns, stakeholders will be incentivized to seek out pools with high pledge levels at or near their saturation levels.